Steve Hsu is a Professor of Theoretical Physics at Michigan State University and cofounder of the company Genomic Prediction.
We go deep into the weeds on how embryo selection can make babies healthier and smarter.

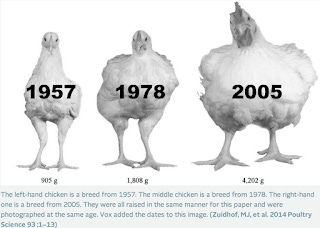
Watch on YouTube. Listen on Apple Podcasts, Spotify, or any other podcast platform.


Read the full transcript here.
Follow Steve on Twitter. Follow me on Twitter for updates on future episodes.
Timestamps
(0:00:14) - Feynman’s advice on picking up women
(0:11:46) - Embryo selection
(0:24:19) - Why hasn't natural selection already optimized humans?
(0:34:13) - Aging
(0:43:18) - First Mover Advantage
(0:53:38) - Genomics in dating
(0:59:20) - Ancestral populations
(1:07:07) - Is this eugenics?
(1:15:08) - Tradeoffs to intelligence
(1:24:25) - Consumer preferences
(1:29:34) - Gwern
(1:33:55) - Will parents matter?
(1:44:45) - Wordcels and shape rotators
(1:56:45) - Bezos and brilliant physicists
(2:09:35) - Elite education
Transcript
Dwarkesh Patel 0:00
Today I have the pleasure of speaking with Steve Hsu. Steve, thanks for coming on the podcast. I'm excited about this.
Steve Hsu 0:04
Hey, it's my pleasure! I'm excited too and I just want to say I've listened to some of your earlier interviews and thought you were very insightful, which is why I was excited to have a conversation with you.
Dwarkesh Patel 0:14
That means a lot for me to hear you say because I'm a big fan of your podcast.
Feynman’s advice on picking up women
Dwarkesh Patel 0:17
So my first question is: “What advice did Richard Feynman give you about picking up girls?”
Steve Hsu 0:24
Haha, wow! So one day in the spring of my senior year, I was walking across campus and saw Feynman coming toward me. We knew each other from various things—it's a small campus, I was a physics major, and he was my hero–– so I'd known him since my first year. He sees me, and he's got this Long Island or New York borough accent and says, "Hey, Hsu!"
I'm like, "Hi, Professor Feynman." We start talking. And he says to me, "Wow, you're a big guy." Of course, I was much bigger back then because I was a linebacker on the Caltech football team. So I was about 200 pounds and slightly over 6 feet tall. I was a gym rat at the time, and I was much bigger than him. He said, "Steve, I got to ask you something." Feynman was born in 1918, so he's not from the modern era. He was going through graduate school when the Second World War started. So, he couldn't understand the concept of a health club or a gym. This was the 80s and was when Gold's Gym was becoming a world national franchise. There were gyms all over the place like 24-Hour Fitness. But, Feynman didn't know what it was.
He's a fascinating guy. He says to me, "What do you guys do there? Is it just a thing to meet girls? Or is it really for training? Do you guys go there to get buff?" So, I started explaining to him that people are there to get big, but people are also checking out the girls. A lot of stuff is happening at the health club or the weight room. Feynman grills me on this for a long time. And one of the famous things about Feynman is that he has a laser focus. So if there's something he doesn't understand and wants to get to the bottom of it, he will focus on you and start questioning you and get to the bottom of it. That's the way his brain worked. So he did that to me for a while because he didn't understand lifting weights and everything. In the end, he says to me, "Wow, Steve, I appreciate that. Let me give you some good advice."
Then, he starts telling me how to pick up girls—which he's an expert on. He says to me, "I don't know how much girls like guys that are as big as you." He thought it might be a turn-off. "But you know what, you have a nice smile." So that was the one compliment he gave me. Then, he starts to tell me that it's a numbers game. You have to be rational about it. You're at an airport lounge, or you're at a bar. It's Saturday night in Pasadena or Westwood, and you're talking to some girl. He says, "You're never going to see her again. This is your five-minute interaction. Do what you have to do. If she doesn't like you, go to the next one." He also shares some colorful details. But, the point is that you should not care what they think of you. You're trying to do your thing. He did have a reputation at Caltech as a womanizer, and I could go into that too, but I heard all this from the secretaries.
Dwarkesh Patel 4:30
With the students or only the secretaries?
Steve Hsu 4:35
Secretaries! Well mostly secretaries. They were almost all female at that time. He had thought about this a lot and thought of it as a numbers game. The PUA guys (pick-up artists) will say, “Follow the algorithm, and whatever happens, it's not a reflection on your self-esteem. It's just what happened. And you go on to the next one.” That was the advice he was giving me, and he said other things that were pretty standard: Be funny, be confident—just basic stuff.
Steve Hu: But the main thing I remember was the operationalization of it as an algorithm. You shouldn’t internalize whatever happens if you get rejected, because that hurts. When we had to go across the bar to talk to that girl (maybe it doesn’t happen in your generation), it was terrifying. We had to go across the bar and talk to some lady! It’s loud, and you’ve got a few minutes to make your case. Nothing is scarier than walking up to the girl and her friends. Feynman was telling me to train myself out of that. You're never going to see them again; the face space of humanity is so big that you'll probably never re-encounter them again. It doesn't matter. So, do your best.
Dwarkesh Patel 6:06
Yeah, that's interesting because.. I wonder whether he was doing this in the 40’–– like when he was at that age, was he doing this? I don't know what the cultural conventions were at the time. Were there bars in the 40s where you could just go ahead and hit on girls or?
Steve Hsu 6:19
Oh yeah, absolutely. If you read literature from that time, or even a little bit earlier like Hemingway or John O'Hara, they talk about how men and women interacted in bars and stuff in New York City. So, that was much more of a thing back than when compared to your generation. That's what I can’t figure out with my kids! What is going on? How do boys and girls meet these days? Back in the day, the guy had to do all the work. It was the most terrifying thing you could do, and you had to train yourself out of that.
Dwarkesh Patel 6:57
By the way, for the context for the audience, when Feynman says you were a big guy, you were a football player at Caltech, right? There's a picture of you on your website, maybe after college or something, but you look pretty ripped. Today, it seems more common because of the gym culture. But I don’t know about back then. I don't know how common that body physique was.
Steve Hsu 7:24
It’s amazing that you asked this question. I'll tell you a funny story. One of the reasons Feynman found this so weird was because of the way body-building entered the United States. They were regarded as freaks and homosexuals at first. I remember swimming and football in high school (swimming is different because it's international), and in swimming, I picked up a lot of advanced training techniques from the Russians and East Germans. But football was more American and not very international. So our football coach used to tell us not to lift weights when we were in junior high school because it made you slow. “You’re no good if you’re bulky.” “You gotta be fast in football.” Then, something changed around the time I was in high school–the coaches figured it out. I began lifting weights since I was an age group swimmer, like maybe age 12 or 14. Then, the football coaches got into it mainly because the University of Nebraska had a famous strength program that popularized it.
At the time, there just weren't a lot of big guys. The people who knew how to train were using what would be considered “advanced knowledge” back in the 80s. For example, they’d know how to do a split routine or squat on one day and do upper body on the next day–– that was considered advanced knowledge at that time. I remember once.. I had an injury, and I was in the trainer's room at the Caltech athletic facility. The lady was looking at my quadriceps. I’d pulled a muscle, and she was looking at the quadriceps right above your kneecap. If you have well-developed quads, you'd have a bulge, a bump right above your cap. And she was looking at it from this angle where she was in front of me, and she was looking at my leg from the front. She's like, “Wow, it's swollen.” And I was like, “That's not the injury. That's my quadricep!” And she was a trainer! So, at that time, I could probably squat 400 pounds. So I was pretty strong and had big legs. The fact that the trainer didn't really understand what well-developed anatomy was supposed to look like blew my mind!
So anyway, we've come a long way. This isn't one of these things where you have to be old to have any understanding of how this stuff evolved over the last 30-40 years.
Dwarkesh Patel 10:13
But, I wonder if that was a phenomenon of that particular time or if people were not that muscular throughout human history. You hear stories of Roman soldiers who are carrying 80 pounds for 10 or 20 miles a day. I mean, there are a lot of sculptures in the ancient world, or not that ancient, but the people look like they have a well-developed musculature.
Steve Hsu 10:34
So the Greeks were very special because they were the first to think about the word gymnasium. It was a thing called the Palaestra, where they were trained in wrestling and boxing. They were the first people who were seriously into physical culture specific training for athletic competition.
Even in the 70s, when I was a little kid, I look back at the guys from old photos and they were skinny. So skinny! The guys who went off and fought World War Two, whether they were on the German side, or the American side, were like 5’8-5’9 weighing around 130 pounds - 140 pounds. They were much different from what modern US Marines would look like. So yeah, physical culture was a new thing. Of course, the Romans and the Greeks had it to some degree, but it was lost for a long time. And, it was just coming back to the US when I was growing up. So if you were reasonably lean (around 200 pounds) and you could bench over 300.. that was pretty rare back in those days.
Embryo selection
Dwarkesh Patel 11:46
Okay, so let's talk about your company Genomic Prediction. Do you want to talk about this company and give an intro about what it is?
Steve Hsu 11:55
Yeah. So there are two ways to introduce it. One is the scientific view. The other is the IVF view. I can do a little of both. So scientifically, the issue is that we have more and more genomic data. If you give me the genomes of a bunch of people and then give me some information about each person, ex. Do they have diabetes? How tall are they? What's their IQ score? It’s a natural AI machine learning problem to figure out which features in the DNA variation between people are predictive of whatever variable you're trying to predict.
This is the ancient scientific question of how you relate the genotype of the organism (the specific DNA pattern), to the phenotype (the expressed characteristics of the organism). If you think about it, this is what biology is! We had the molecular revolution and figured out that it’s people's DNA that stores the information which is passed along. Evolution selects on the basis of the variation in the DNA that’s expressed as phenotype, as that phenotype affects fitness/reproductive success. That's the whole ballgame for biology. As a physicist who's trained in mathematics and computation, I'm lucky that I arrived on the scene at a time when we're going to solve this basic fundamental problem of biology through brute force, AI, and machine learning. So that's how I got into this. Now you ask as an entrepreneur, “Okay, fine Steve, you're doing this in your office with your postdocs and collaborators on your computers. What use is it?”
The most direct application of this is in the following setting: Every year around the world, millions of families go through IVF—typically because they're having some fertility issues, and also mainly because the mother is in her 30s or maybe 40s. In the process of IVF, they use hormone stimulation to produce more eggs. Instead of one per cycle, depending on the age of the woman, they might produce anywhere between five to twenty, or even sixty to a hundred eggs for young women who are hormonally stimulated (egg donors).
From there, it’s trivial because men produce sperm all the time. You can fertilize eggs pretty easily in a little dish, and get a bunch of embryos that grow. They start growing once they're fertilized. The problem is that if you're a family and produce more embryos than you’re going to use, you have the embryo choice problem. You have to figure out which embryo to choose out of say, 20 viable embryos.
The most direct application of the science that I described is that we can now genotype those embryos from a small biopsy. I can tell you things about the embryos. I could tell you things like your fourth embryo being an outlier. For breast cancer risk, I would think carefully about using number four. Number ten is an outlier for cardiovascular disease risk. You might want to think about not using that one. The other ones are okay. So, that’s what genomic prediction does. We work with 200 or 300 different IVF clinics in six continents.
Dwarkesh Patel 15:46
Yeah, so the super fascinating thing about this is that the diseases you talked about—or at least their risk profiles—are polygenic. You can have thousands of SNPs (single nucleotide polymorphisms) determining whether you will get a disease. So, I'm curious to learn how you were able to transition to this space and how your knowledge of mathematics and physics was able to help you figure out how to make sense of all this data.
Steve Hsu 16:16
Yeah, that's a great question. So again, I was stressing the fundamental scientific importance of all this stuff. If you go into a slightly higher level of detail—which you were getting at with the individual SNPs, or polymorphisms—there are individual locations in the genome, where I might differ from you, and you might differ from another person. Typically, each pair of individuals will differ at a few million places in the genome—and that controls why I look a little different than you
A lot of times, theoretical physicists have a little spare energy and they get tired of thinking about quarks or something. They want to maybe dabble in biology, or they want to dabble in computer science, or some other field. As theoretical physicists, we always feel, “Oh, I have a lot of horsepower, I can figure a lot out.” (For example, Feynman helped design the first parallel processors for thinking machines.) I have to figure out which problems I can make an impact on because I can waste a lot of time. Some people spend their whole lives studying one problem, one molecule or something, or one biological system. I don't have time for that, I'm just going to jump in and jump out. I'm a physicist. That's a typical attitude among theoretical physicists.
So, I had to confront sequencing costs about ten years ago because I knew the rate at which they were going down. I could anticipate that we’d get to the day (today) when millions of genomes with good phenotype data became available for analysis. A typical training run might involve almost a million genomes or half a million genomes. The mathematical question then was: What is the most effective algorithm given a set of genomes and phenotype information to build the best predictor? This can be boiled down to a very well-defined machine learning problem. It turns out, for some subset of algorithms, there are theorems— performance guarantees that give you a bound on how much data you need to capture almost all of the variation in the features. I spent a fair amount of time, probably a year or two, studying these very famous results, some of which were proved by a guy named Terence Tao, a Fields medalist.
These are results on something called compressed sensing: a penalized form of high dimensional regression that tries to build sparse predictors. Machine learning people might notice L1-penalized optimization. The very first paper we wrote on this was to prove that using accurate genomic data and these very abstract theorems in combination could predict how much data you need to “solve” individual human traits. We showed that you would need at least a few hundred thousand individuals and their genomes and their heights to solve for height as a phenotype. We proved that in a paper using all this fancy math in 2012. Then around 2017, when we got a hold of half a million genomes, we were able to implement it in practical terms and show that our mathematical result from some years ago was correct. The transition from the low performance of the predictor to high performance (which is what we call a “phase transition boundary” between those two domains) occurred just where we said it was going to occur. Some of these technical details are not understood even by practitioners in computational genomics who are not quite mathematical. They don't understand these results in our earlier papers and don't know why we can do stuff that other people can't, or why we can predict how much data we'll need to do stuff. It's not well-appreciated, even in the field.
But when the big AI in our future in the singularity looks back and says, “Hey, who gets the most credit for this genomics revolution that happened in the early 21st century?” they're going to find these papers on the archive where we proved this was possible, and how five years later, we actually did it. Right now, it's under-appreciated, but the future AI––that Roko's Basilisk AI–will look back and will give me a little credit for it.
Dwarkesh Patel 21:03
Yeah, I was a little interested in this a few years ago. At that time, I looked into how these polygenic risk scores were calculated. Basically, you find the correlation between the phenotype and the alleles that correlate with it. You add up how many copies of these alleles you have, what the correlations are, and you do a weighted sum of that. So that seemed very simple, especially in an era where we have all this machine learning, but it seems like they're getting good predictive results out of this concept. So, what is the delta between how good you can go with all this fancy mathematics versus a simple sum of correlations?
Steve Hsu 21:43
You're right that the ultimate models that are used when you've done all the training and when the dust settles, are straightforward. They’re pretty simple and have an additive structure. Basically, I either assign a nonzero weight to this particular region in the genome, or I don't. Then, I need to know what the weighting is, but then the function is a linear function or additive function of the state of your genome at some subset of positions. The ultimate model that you get is straightforward. Now, if you go back ten years, when we were doing this, there were lots of claims that it was going to be super nonlinear—that it wasn't going to be additive the way I just described it. There were going to be lots of interaction terms between regions. Some biologists are still convinced that's true, even though we already know we have predictors that don't have interactions.
The other question, which is more technical, is whether in any small region of your genome, the state of the individual variants is highly correlated because you inherit them in chunks. You need to figure out which one you want to use. You don't want to activate all of them because you might be overcounting. So that's where these L-1 penalization sparse methods force the predictor to be sparse. That is a key step. Otherwise, you might overcount. If you do some simple regression math, you might have 10-10 different variants close by that have roughly the same statistical significance.
But, you don't know which one of those tends to be used, and you might be overcounting effects or undercounting effects. So, you end up doing a high-dimensional optimization, where you grudgingly activate an SNP when the signal is strong enough. Once you activate that one, the algorithm has to be smart enough to penalize the other ones nearby and not activate them because you're over-counting effects if you do that. There's a little bit of subtlety in it. But, the main point you made is that the ultimate predictors, which are very simple and addictive—sum over effect sizes and time states—work well. That’s related to a deep statement about the additive structure of the genetic architecture of individual differences.
In other words, it's weird that the ways that I differ from you are merely just because I have more of something or you have less of something. It’s not like these things are interacting in some incredibly understandable way. That's a deep thing—which is not appreciated that much by biologists yet. But over time, they'll figure out something interesting here.
Why hasn’t natural selection already optimized humans?
Dwarkesh Patel 24:19
Right. I thought that was super fascinating, and I commented on that on Twitter. What is interesting about that is two things. One is that you have this fascinating evolutionary argument about why that would be the case that you might want to explain. The second is that it makes you wonder if becoming more intelligent is just a matter of turning on certain SNPs. It's not a matter of all this incredible optimization being like solving a sudoku puzzle or anything. If that's the case, then why hasn't the human population already been selected to be maxed out on all these traits if it's just a matter of a bit flip?
Steve Hsu 25:00
Okay, so the first issue is why is this genetic architecture so surprisingly simple? Again, we didn't know it would be simple ten years ago. So when I was checking to see whether this was a field that I should go into depending on our capabilities to make progress, we had to study the more general problem of the nonlinear possibilities. But eventually, we realized that most of the variance would probably be captured in an additive way. So, we could narrow down the problem quite a bit. There are evolutionary reasons for this. There’s a famous theorem by Fisher, the father of population genetics (aka. frequentist statistics). Fisher proved something called Fisher's Fundamental Theorem of Natural Selection, which says that if you impose some selection pressure on a population, the rate at which that population responds to the selection pressure (let’s say it’s the bigger rats that out-compete, the smaller rats) then at what rate does the rat population start getting bigger?
He showed that it's the additive variants that dominate the rate of evolution. It's easy to understand why if it's a nonlinear mechanism, you need to make the rat bigger. When you sexually reproduce, and that gets chopped apart, you might break the mechanism. Whereas, if each short allele has its own independent effect, you can inherit them without worrying about breaking the mechanisms. It was well known among a tiny theoretical population of biologists that adding variants was the dominant way that populations would respond to selection. That was already known. The other thing is that humans have been through a pretty tight bottleneck, and we're not that different from each other. It's very plausible that if I wanted to edit a human embryo and make it into a frog, then there are all kinds of subtle nonlinear things I’d have to do. But all those identical nonlinear complicated subsystems are fixed in humans. You have the same system as I do. You have the not human, not frog or ape, version of that region of DNA, and so do I. But the small ways we differ are mostly little additive switches. That's this deep scientific discovery from over the last 5-10 years of work in this area.
Now, you were asking about why evolution hasn't completely “optimized” all traits in humans already. I don't know if you’ve ever done deep learning or high-dimensional optimization, but in that high-dimensional space, you're often moving on a slightly-tilted surface. So, you're getting gains, but it's also flat. Even though you scale up your compute or data size by order of magnitude, you don't move that much farther. You get some gains, but you're never really at the global max of anything in these high-dimensional spaces. I don't know if that makes sense to you. But it's pretty plausible to me that two things are important here. One is that evolution has not had that much time to optimize humans. The environment that humans live in changed radically in the last 10,000 years. For a while, we didn't have agriculture, and now we have agriculture. Now, we have a swipe left if you want to have sex tonight. The environment didn't stay fixed. So, when you say fully optimized for the environment, what do you mean?
The ability to diagonalize matrices might not have been very adaptive 10,000 years ago. It might not even be adaptive now. But anyway, it's a complicated question that one can't reason naively about. “If God wanted us to be 10 feet tall, we'd be 10 feet tall.” Or “if it's better to be smart, my brain would be *this* big or something.” You can't reason naively about stuff like that.
Dwarkesh Patel 29:04
I see. Yeah.. Okay. So I guess it would make sense then that for example, with certain health risks, the thing that makes you more likely to get diabetes or heart disease today might be… I don't know what the pleiotropic effect of that could be. But maybe that's not that important one year from now.
Steve Hsu 29:17
Let me point out that most of the diseases we care about now—not the rare ones, but the common ones—manifest when you're 50-60 years old. So there was never any evolutionary advantage of being super long-lived. There's even a debate about whether the grandparents being around to help raise the kids lifts the fitness of the family unit. But, most of the time in our evolutionary past, humans just died fairly early. So, many of these diseases would never have been optimized against evolution. But, we see them now because we live under such good conditions, we can regulate people over 80 or 90 years.
Dwarkesh Patel 29:57
Regarding the linearity and additivity point, I was going to make the analogy that– and I'm curious if this is valid– but when you're programming, one thing that's good practice is to have all the implementation details in separate function calls or separate programs or something, and then have your main loop of operation just be called different functions like, “Do this, do that”, so that you can easily comment stuff away or change arguments. This seemed very similar to that where by turning these names on and off, you can change what the next offering will be. And, you don't have to worry about actually implementing whatever the underlying mechanism is.
Steve Hsu 30:41
Well, what you said is related to what Fisher proved in his theorems. Which is that, if suddenly, it becomes advantageous to have X, (like white fur instead of black fur) or something, it would be best if there were little levers that you could move somebody from black fur to white fur continuously by modifying those switches in an additive way. It turns out that for sexually reproducing species where the DNA gets scrambled up in every generation, it's better to have switches of that kind. The other point related to your software analogy is that there seem to be modular, fairly modular things going on in the genome.
When we looked at it, we were the first group to have, initially, 20 primary disease conditions we had decent predictors for. We started looking carefully at just something as trivial as the overlap of my sparsely trained predictor. It turns on and uses *these* features for diabetes, but it uses *these* features for schizophrenia. It’s the stupidest metric, it’s literally just how much overlap or variance accounted for overlap is there between pairs of disease conditions. It's very modest. It's the opposite of what naive biologists would say when they talk about pleiotropy.
They're just disjoint! Disjoint regions of your genome that govern certain things. And why not? You have 3 billion base pairs—there's a lot you can do in there. There's a lot of information there. If you need 1000 to control diabetes risk, I estimated you could easily have 1000 roughly independent traits that are just disjoint in their genetic dependencies. So, if you think about D&D, your strength, decks, wisdom, intelligence, and charisma—those are all disjoint. They're all just independent variables. So it's like a seven-dimensional space that your character lives in. Well, there's enough information in the few million differences between you and me. There's enough for a 1000-dimensional space of variation.
“Oh, how considerable is your spleen?” My spleen is a little bit smaller, yours is a little bit bigger - that can vary independently of your IQ. Oh, it's a big surprise. The size of your spleen can vary independently of the size of your big toe. If you do information theory, there are about 1000 different parameters, and I can vary independently with the number of variants I have between you and me. Because you understand some information theory, it’s trivial to explain, but try explaining to a biologist and you won't get very far.
Dwarkesh Patel 33:27
Yeah, yeah, do the log two of the number of.. is that basically how you do it? Yeah.
Steve Hsu 33:33
Okay. That's all it is. I mean, it's in our paper. We look at how many variants typically account for most of the variation for any of these major traits, and then imagine that they're mostly disjoint. Then it’s just all about: how many variants do you need to independently vary 1000 traits? Well, a few million differences between you and me are enough. It's very trivial math. Once you understand the base and how to reason about information theory, then it's very trivial. But, it ain’t trivial for theoretical biologists, as far as I can tell.
Aging
Dwarkesh Patel 34:13
But the result is so interesting because I remember reading in The Selfish Gene that, as he (Dawkins) hypothesizes that the reason we could be aging is an antagonistic clash. There's something that makes you healthier when you're young and fertile that makes you unhealthy when you're old. Evolution would have selected for such a trade-off because when you're young and fertile, evolution and your genes care about you. But, if there's enough space in the genome —where these trade-offs are not necessarily necessary—then this could be a bad explanation for aging, or do you think I'm straining the analogy?
Steve Hsu 34:49
I love your interviews because the point you're making here is really good. So Dawkins, who is an evolutionary theorist from the old school when they had almost no data—you can imagine how much data they had compared to today—he would tell you a story about a particular gene that maybe has a positive effect when you're young, but it makes you age faster.
So, there's a trade-off. We know about things like sickle cell anemia. We know stories about that. No doubt, some stories are true about specific variants in your genome. But that's not the general story. The general story you only discovered in the last five years is that thousands of variants control almost every trait and those variants tend to be disjoint from the ones that control the other trait. They weren't wrong, but they didn't have the big picture.
Dwarkesh Patel 35:44
Yeah, I see. So, you had this paper, it had polygenic, health index, general health, and disease risk.. You showed that with ten embryos, you could increase disability-adjusted life years by four, which is a massive increase if you think about it. Like what if you could live four years longer and in a healthy state?
Steve Hsu 36:05
Yeah, what's the value of that? What would you pay to buy that for your kid?
Dwarkesh Patel 36:08
Yeah. But, going back to the earlier question about the trade-offs and why this hasn't already been selected for, if you're right and there's no trade-off to do this, just living four years older (even if that's beyond your fertility) just being a grandpa or something seems like an unmitigated good. So why hasn’t this kind of assurance hasn't already been selected for?
Steve Hsu 36:35
I’m glad you're asking about these questions because these are things that people are very confused about, even in the field. First of all, let me say that when you have a trait that's controlled by 10,000 variants (eg. height is controlled by an order of 10,000 variants and probably cognitive ability a little bit more), the square root of 10,000 is 100. So, if I could come to this little embryo, and I want to give it one extra standard deviation of height, I only need to edit 100. I only need to flip 100 minus variance to plus variance. These are very rough numbers. But, one standard deviation is the square root of “n”. If I flip a coin “n” times, I want a better outcome in terms of the number of ratio heads to tails. I want to increase it by one standard deviation. I only need to flip the square root of “n” heads because if you flip a lot, you will get a narrow distribution that peaks around half and the width of that distribution is the square root of “n”.
Once I tell you, “Hey, your height is controlled by 10,000 variants, and I only need to flip 100 genetic variants to make you one standard deviation for a male,” (that would be three inches tall, two and a half or three inches taller), you suddenly realize, “Wait a minute, there are a lot of variants up for grabs there. If I could flip 500 variants in your genome, I would make you five standard deviations taller, you'd be seven feet tall.” I didn't even have to do that much work, and there's a lot more variation where that came from. I could have flipped even more because I only flipped 500 out of 10,000, right? So, there's this quasi-infinite well of variation that evolution or genetic engineers could act on. Again, the early population geneticists who bred corn and animals know this. This is something they explicitly know about because they've done calculations.
Interestingly, human geneticists who are mainly concerned with diseases and stuff, are often unfamiliar with the math that the animal breeders already know. You might be interested to know that the milk you drink comes from heavily genetically-optimized cows bred artificially using almost exactly the same technologies that we use for genomic prediction. But they're doing it to optimize milk production and stuff like this. So there is a big well of variance. It's a consequence of the trait's polygenicity. On the longevity side of things, it does look like people could “be engineered” to live much longer by flipping the variants that make the risk for diseases that shorten your life. The question is then, “Why didn't evolution give us life spans of thousands of years?” People in the Bible used to live for thousands of years. Why don't we? I mean, *chuckles* that probably didn’t happen. But the question is, you have this very high dimensional space, and you have a fitness function. How big is the slope in a particular direction of that fitness function? How much more successful reproductively would Joe caveman have been if he lived to be 150 instead of only 100 or something? There just hasn't been enough time to explore this super high-dimensional space. That's the actual answer. But now, we have the technology, and we're going to fucking explore it fast. That's the point that the big lightbulb should go off. We’re mapping this space out now. Pretty confident in 10 years or so, with the CRISPR gene editing technologies will be ready for massively multiplexed edits. We'll start navigating in this high-dimensional space as much as we like. So that's the more long-term consequence of the scientific insights.
Dwarkesh Patel 40:53
Yeah, that's super interesting. What do you think will be the plateau for a trait of how long you’ll live? With the current data and techniques, do you think it could be significantly greater than that?
Steve Hsu 41:05
We did a simple calculation—which amazingly gives the correct result. This polygenic predictor that we built (which isn't perfect yet but will improve as we gather more data) is used in selecting embryos today. If you asked, out of a billion people, “What's the best person typically, what would their score be on this index and then how long would they be predicted to live?”’ It's about 120 years. So it's spot on.
One in a billion types of person lives to be 120 years old. How much better can you do? Probably a lot better. I don't want to speculate, but other nonlinear effects, things that we're not taking into account will start to play a role at some point. So, it's a little bit hard to estimate what the true limiting factors will be.
But one super robust statement, and I'll stand by it, debate any Nobel Laureate in biology who wants to discuss it even, is that there are many variants available to be selected or edited. There's no question about that. That's been established in animal breeding in plant breeding for a long time now. If you want a chicken that grows to be *this* big, instead of *this* big, you can do it. You can do it if you want a cow that produces 10 times or 100 times more milk than a regular cow. The egg you ate for breakfast this morning, those bio-engineered chickens that lay almost an egg a day… A chicken in the wild lays an egg a month. How the hell did we do that? By genetic engineering. That's how we did it.
Dwarkesh Patel 42:51
Yeah. That was through brute artificial selection. No fancy machine learning there.
Steve Hsu 42:58
Last ten years, it's gotten sophisticated machine learning genotyping of chickens. Artificial insemination, modeling of the traits using ML last ten years. For cow breeding, it's done by ML.
First Mover Advantage
Dwarkesh Patel 43:18
I had no idea. That's super interesting. So, you mentioned that you're accumulating data and improving your techniques over time, is there a first mover advantage to a genomic prediction company like this? Or is it whoever has the newest best algorithm for going through the biobank data?
Steve Hsu 44:16
That's another super question. For the entrepreneurs in your audience, I would say in the short run, if you ask what the valuation of GPB should be? That's how the venture guys would want me to answer the question. There is a huge first mover advantage because they're important in the channel relationships between us and the clinics. Nobody will be able to get in there very easily when they come later because we're developing trust and an extensive track record with clinics worldwide—and we're well-known.
So could 23andme or some company with a huge amount of data—if they were to get better AI/ML people working on this—blow us away a little bit and build better predictors because they have much more data than we do? Possibly, yes. Now, we have had core expertise in doing this work for years that we're just good at it. Even though we don't have as much data as 23andme, our predictors might still be better than theirs.
I'm out there all the time, working with biobanks all around the world. I don't want to say all the names, but other countries are trying to get my hands on as much data as possible.
But, there may not be a lasting advantage beyond the actual business channel connections to that particular market. It may not be a defensible, purely scientific moat around the company. We have patents on specific technologies about how to do genotyping or error correction on the embryo, DNA, and stuff like this. We do have patents on stuff like that. But this general idea of who will best predict human traits from DNA? It's unclear who's going to be the winner in that race. Maybe it'll be the Chinese government in 50 years? Who knows?
Dwarkesh Patel 46:13
Yeah, that's interesting. If you think about a company Google, theoretically, it's possible that you could come up with a better algorithm than PageRank and beat them. But it seems like the engineer at Google is going to come up with whatever edge case or whatever improvement is possible.
Steve Hsu 46:28
That's exactly what I would say. PageRank is deprecated by now. But, even if somebody else comes up with a somewhat better algorithm if they have a little bit more data, if you have a team doing this for a long time and you're focused and good, it's still tough to beat you, especially if you have a lead in the market.
Dwarkesh Patel 46:50
So, are you guys doing the actual biopsy? Or is it just that they upload the genome, and you're the one processing just giving recommendations? Is it an API call, basically?
Steve Hsu 47:03
It's great, I love your question. It is totally standard. Every good IVF clinic in the world regularly takes embryo biopsies. So that's standard. There’s a lab tech doing that. Okay. Then, they take the little sample, put it on ice, and ship it. The DNA as a molecule is exceptionally robust and stable. My other startup solves crimes that are 100 years old from DNA that we get from some semen stain on some rape victim, serial killer victims bra strap, we've done stuff that.
Dwarkesh Patel 47:41
Jack the Ripper, when are we going to solve that mystery?
Steve Hsu 47:44
If they can give me samples, we can get into that. For example, we just learned that you could recover DNA pretty well if someone licks a stamp and puts it on their correspondence. If you can do Neanderthals, you can do a lot to solve crimes. In the IVF workflow, our lab, which is in New Jersey, can service every clinic in the world because they take the biopsy, put it in a standard shipping container, and send it to us. We’re actually genotyping DNA in our lab, but we've trained a few of the bigger clinics to do the genotyping on their site. At that point, they upload some data into the cloud, and then they get back some stuff from our platform. And at that point, it's going to be the whole world, every human who wants their kid to be healthy and get the best they can– that data is going to come up to us, and the report is going to come back down to their IVF physician.
Dwarkesh Patel 48:46
Which is great if you think that there's a potential that this technology might get regulated in some way, you could go to Mexico or something, have them upload the genome (you don't care what they upload it from), and then get the recommendations there.
Steve Hsu 49:05
I think we’re going to evolve to a point where we are going to be out of the wet part of this business and only in the cloud and bit part of this business. No matter where it is, the clinics are going to have a sequencer, which is *this* big, and their tech is going to quickly upload and retrieve the report for the physician three seconds later. Then, the parents are going to look at it on their phones or whatever. We’re basically there with some clinics. It’s going to be tough to regulate because it’s just this. You have the bits, and you’re in some repressive, terrible country that doesn’t allow you to select for some special traits that people are nervous about, but you can upload it to some vendor that’s in Singapore or some free country, and they give you the report back.
Doesn’t have to be us, we don’t do the edgy stuff. We only do the health-related stuff right now. But, if you want to know how tall this embryo is going to be… I’ll tell you a mind-blower! When you do face recognition in AI, you're mapping someone's face into a parameter space on the order of hundreds of parameters, each of those parameters is super heritable.
In other words, if I take two twins and photograph them, and the algorithm gives me the value of that parameter for twin one and two, they're very close. That's why I can't tell the two twins apart, and face recognition can ultimately tell them apart if it’s really good system. But you can conclude that almost all these parameters are identical for those twins. So it's highly heritable.
We're going to get to a point soon where I can do the inverse problem where I have your DNA and I predict each of those parameters in the face recognition algorithm and then reconstruct the face. If I say that when this embryo will be 16, that is what she will look like. When she's 32, this is what she's going to look like. I'll be able to do that, for sure. It's only an AI/ML problem right now. But basic biology is clearly going to work. So then you're going to be able to say, “Here's a report. Embryo four is so cute.” Before, we didn't know we wouldn't do that, but it will be possible.
Dwarkesh Patel 51:37
Before we get married, you'll want to see what their genotype implies about their faces' longevity. It's interesting that you hear stories about these cartel leaders who will get plastic surgery or something to evade the law, you could have a check where you look at a lab and see if it matches the face you would have had five years ago when they caught you on tape.
Steve Hsu 52:02
This is a little bit back to old-school Gattaca, but you don't even need the face! You can just take a few molecules of skin cells and phenotype them and know exactly who they are. I've had conversations with these spooky Intel folks. They're very interested in, “Oh, if some Russian diplomat comes in, and we think he's a spy, but he's with the embassy, and he has a coffee with me, and I save the cup and send it to my buddy at Langley, can we figure out who this guy is? And that he has a daughter who's going to Chote? Can do all that now.
Dwarkesh Patel 52:49
If that's true, then in the future, world leaders will not want to eat anything or drink. They'll be wearing a hazmat suit to make sure they don't lose a hair follicle.
Steve Hsu 53:04
The next time Pelosi goes, she will be in a spacesuit if she cares. Or the other thing is, they're going to give it. They're just going to be, “Yeah, my DNA is everywhere. If I'm a public figure, I can't track my DNA. It's all over.”
Dwarkesh Patel 53:17
But the thing is, there's so much speculation that Putin might have cancer or something. If we have his DNA, we can see his probability of having cancer at age 70, or whatever he is, is 85%. So yeah, that’d be a very verified rumor. That would be interesting.
Steve Hsu 53:33
I don't think that would be very definitive. I don't think we'll reach that point where you can say that Putin has cancer because of his DNA—which I could have known when he was an embryo. I don't think it's going to reach that level. But, we could say he is at high risk for a type of cancer.
Genomics in dating
Dwarkesh Patel 53:49
In 50 or 100 years, if the majority of the population is doing this, and if the highly heritable diseases get pruned out of the population, does that mean we'll only be left with lifestyle diseases? So, you won't get breast cancer anymore, but you will still get fat or lung cancer from smoking?
Steve Hsu 54:18
It's hard to discuss the asymptotic limit of what will happen here. I'm not very confident about making predictions like that. It could get to the point where everybody who's rich or has been through this stuff for a while, (especially if we get the editing working) is super low risk for all the top 20 killer diseases that have the most life expectancy impact. Maybe those people live to be 300 years old naturally. I don't think that's excluded at all. So, that's within the realm of possibility. But it's going to happen for a few lucky people like Elon Musk before it happens for shlubs like you and me.
There are going to be very angry inequality protesters about the Trump grandchildren, who, models predict will live to be 200 years old. People are not going to be happy about that.
Dwarkesh Patel 55:23
So interesting. So, one way to think about these different embryos is if you're producing multiple embryos, and you get to select from one of them, each of them has a call option, right? Therefore, you probably want to optimize for volatility as much, or if not more than just the expected value of the trait. So, I'm wondering if there are mechanisms where you can increase the volatility in meiosis or some other process. You just got a higher variance, and you can select from the tail better.
Steve Hsu 55:55
Well, I'll tell you something related, which is quite amusing. So I talked with some pretty senior people at the company that owns all the dating apps. So you can look up what company this is, but they own Tinder and Match. They’re kind of interested in perhaps including a special feature where you upload your genome instead of Tinder Gold / Premium. And when you match- you can talk about how well you match the other person based on your genome. One person told me something shocking. Guys lie about their height on these apps.
Dwarkesh Patel 56:41
I’m shocked, truly shocked hahaha.
Steve Hsu 56:45
Suppose you could have a DNA-verified height. It would prevent gross distortions if someone claims they're 6’2 and they’re 5’9. The DNA could say that's unlikely. But no, the application to what you were discussing is more like, “Let's suppose that we're selecting on intelligence or something. Let's suppose that the regions where your girlfriend has all the plus stuff are complementary to the regions where you have your plus stuff. So, we could model that and say, because of the complementarity structure of your genome in the regions that affect intelligence, you're very likely to have some super intelligent kids way above your, the mean of your you and your girlfriend's values. So, you could say things like it being better for you to marry that girl than another. As long as you go through embryo selection, we can throw out the bad outliers. That's all that's technically feasible.
It's true that one of the earliest patent applications, they'll deny it now. What's her name? Gosh, the CEO of 23andme…Wojcicki, yeah. She'll deny it now. But, if you look in the patent database, one of the very earliest patents that 23andme filed when they were still a tiny startup was about precisely this: Advising parents about mating and how their kids would turn out and stuff like this. We don't even go that far in GP, we don't even talk about stuff like that, but they were thinking about it when they founded 23andme.
Dwarkesh Patel 58:38
That is unbelievably interesting. By the way, this just occurred to me—it's supposed to be highly heritable, especially people in Asian countries, who have the experience of having grandparents that are much shorter than us, and then parents that are shorter than us, which suggests that the environment has a big part to play in it malnutrition or something. So how do you square that our parents are often shorter than us with the idea that height is supposed to be super heritable.
Steve Hsu 59:09
Another great observation. So the correct scientific statement is that we can predict height for people who will be born and raised in a favorable environment. In other words, if you live close to a McDonald's and you're able to afford all the food you want, then the height phenotype becomes super heritable because the environmental variation doesn't matter very much. But, you and I both know that people are much smaller if we return to where our ancestors came from, and also, if you look at how much food, calories, protein, and calcium they eat, it's different from what I ate and what you ate growing up. So we're never saying the environmental effects are zero. We're saying that for people raised in a particularly favorable environment, maybe the genes are capped on what can be achieved, and we can predict that. In fact, we have data from Asia, where you can see much bigger environmental effects. Age affects older people, for fixed polygenic scores on the trait are much shorter than younger people.
Ancestral populations
Dwarkesh Patel 1:00:31
Oh, okay. Interesting. That raises the next question I was about to ask: how applicable are these scores across different ancestral populations?
Steve Hsu 1:00:44
Huge problem is that most of the data is from Europeans. What happens is that if you train a predictor in this ancestry group and go to a more distant ancestry group, there's a fall-off in the prediction quality. Again, this is a frontier question, so we don't know the answer for sure. But many people believe that there's a particular correlational structure in each population, where if I know the state of this SNP, I can predict the state of these neighboring SNPs. That is a product of that group's mating patterns and ancestry. Sometimes, the predictor, which is just using statistical power to figure things out, will grab one of these SNPs as a tag for the truly causal SNP in there. It doesn't know which one is genuinely causal, it is just grabbing a tag, but the tagging quality falls off if you go to another population (eg. This was a very good tag for the truly causal SNP in the British population. But it's not so good a tag in the South Asian population for the truly causal SNP, which we hypothesize is the same).
It's the same underlying genetic architecture in these different ancestry groups. We don't know if that's a hypothesis. But even so, the tagging quality falls off. So my group spent a lot of our time looking at the performance of predictor training population A, and on distant population B, and modeling it trying to figure out trying to test hypotheses as to whether it's just the tagging decay that’s responsible for most of the faults. So all of this is an area of active investigation. It'll probably be solved in five years. The first big biobanks that are non-European are coming online. We're going to solve it in a number of years.
Dwarkesh Patel 1:02:38
Oh, what does the solution look like? Unless you can identify the causal mechanism by which each SNP is having an effect, how can you know that something is a tag or whether it's the actual underlying switch?
Steve Hsu 1:02:54
The nature of reality will determine how this is going to go. So we don't truly know if the innate underlying biology is true. This is an amazing thing. People argue about human biodiversity and all this stuff, and we don't even know whether these specific mechanisms that predispose you to be tall or have heart disease are the same in these different ancestry groups. We assume that it is, but we don't know that. As we get further away to Neanderthals or Homo Erectus, you might see that they have a slightly different architecture than we do.
But let's assume that the causal structure is the same for South Asians and British people. Then it's a matter of improving the tags. How do I know if I don't know which one is causal? What do I mean by improving the tags? This is a machine learning problem. If there's an SNP, which is always coming up as very significant when I use it across multiple ancestry groups, maybe that one's casual. As I vary the tagging correlations in the neighborhood of that SNP, I always find that that one is the intersection of all these different sets, making me think that one's going to be causal. That's a process we're engaged in now—trying to do that. Again, it's just a machine learning problem. But we need data. That's the main issue.
Dwarkesh Patel 1:04:32
I was hoping that wouldn't be possible because one way we might go about this research is that it itself becomes taboo or causes other sorts of bad social consequences if you can definitively show that on certain traits, there are differences between ancestral populations, right?
So, I was hoping that maybe there was an evasion button where we can't say because they're just tags, and the tags might be different between different ancestral populations. But with machine learning, we’ll know.
Steve Hsu 1:04:59
That's the situation we're in now, where you have to do some fancy analysis if you want to claim that Italians have lower height potential than Nordics—which is possible. There's been a ton of research about this because there are signals of selection. The alleles, which are activated in height predictors, look like they've been under some selection between North and South Europe over the last 5000 years for whatever reason. But, this is a thing debated by people who study molecular evolution.
But suppose it's true, okay? That would mean that when we finally get to the bottom of it, we find all the causal loci for height, and the average value for the Italians is lower than that for those living in Stockholm. That might be true. People don't get that excited? They get a little bit excited about height. But they would get really excited if this were true for some other traits, right?
Suppose the causal variants affecting your level of extraversion are systematic, that the average value of those weighed the weighted average of those states is different in Japan versus Sicily. People might freak out over that. I'm supposed to say that's obviously not true. How could it possibly be true? There hasn't been enough evolutionary time for those differences to arise. After all, it's not possible that despite what looks to be the case for height over the last 5000 years in Europe, no other traits could have been differentially selected over the last 5000 years. That's the dangerous thing. Few people understand this field well enough to understand what you and I just discussed and are so alarmed by it that they're just trying to suppress everything. Most of them don't follow it at this technical level that you and I are just discussing. So, they're somewhat instinctively negative about it, but they don't understand it very well.
Dwarkesh Patel 1:07:19
That's good to hear. You see this pattern that by the time that somebody might want to regulate or in some way interfere with some technology or some information, it already has achieved wide adoption. You could argue that that's the case with crypto today. But if it's true that a bunch of IVF clinics worldwide are using these scores to do selection and other things, by the time people realize the implications of this data for other kinds of social questions, this has already been an existing consumer technology.
Is this eugenics?
Steve Hsu 1:07:58
That's true, and the main outcry will be if it turns out that there are massive gains to be had, and only the billionaires are getting them. But that might have the consequence of causing countries to make this free part of their national health care system. So Denmark and Israel pay for IVF. For infertile couples, it's part of their national health care system. They're pretty aggressive about genetic testing. In Denmark, one in 10 babies are born through IVF. It's not clear how it will go. But we're in for some fun times. There's no doubt about that.
Dwarkesh Patel 1:08:45
Well, one way you could go is that some countries decided to ban it altogether. And another way it could go is if countries decided to give everybody free access to it. If you had to choose between the two, you would want to go for the second one. Which would be the hope. Maybe only those two are compatible with people's moral intuitions about this stuff.
Steve Hsu 1:09:10
It’s very funny because most wokeist people today hate this stuff. But, most progressives like Margaret Sanger, or anybody who was the progressive intellectual forebears of today's wokeist, in the early 20th century, were all that we would call today in Genesis because they were like, “Thanks to Darwin, we now know how this all works. We should take steps to keep society healthy and (not in a negative way where we kill people we don't like, but we should help society do healthy things when they reproduce and have healthy kids).” Now, this whole thing has just been flipped over among progressives.
Dwarkesh Patel 1:09:52
Even in India, less than 50 years ago, Indira Gandhi, she's on the left side of India's political spectrum. She was infamous for putting on these forced sterilization programs. Somebody made an interesting comment about this where they were asked, “Oh, is it true that history always tilts towards progressives? And if so, isn't everybody else doomed? Aren't their views doomed?”
The person made a fascinating point: whatever we consider left at the time tends to be winning. But what is left has changed a lot over time, right? In the early 20th century, prohibition was a left cause. It was a progressive cause, and that changed, and now the opposite is the left cause. But now, legalizing pot is progressive. Exactly. So, if Conquest’s second law is true, and everything tilts leftover time, just change what is left is, right? That's the solution.
Steve Hsu 1:10:59
No one can demand that any of these woke guys be intellectually self-consistent, or even say the same things from one year to another. But one could wonder what they think about these literally Communist Chinese. They’re recycling huge parts of their GDP to help the poor and the southern stuff. Medicine is free, education is free, right? They're clearly socialists, and literally communists. But in Chinese, the Chinese characters for eugenics is a positive thing. It means healthy production.
But more or less, the whole viewpoint on all this stuff is 180 degrees off in East Asia compared to here, and even among the literal communists—so go figure.
Dwarkesh Patel 1:11:55
Yeah, very based. So let's talk about one of the traits that people might be interested in potentially selecting for: intelligence. What is the potential for us to acquire the data to correlate the genotype with intelligence?
Steve Hsu 1:12:15
Well, that's the most personally frustrating aspect of all of this stuff. If you asked me ten years ago when I started doing this stuff what were we going to get, everything was gone. On the optimistic side of what I would have predicted, so everything's good. Didn't turn out to be interactively nonlinear, or it didn't turn out to be interactively pleiotropic. All these good things, —which nobody could have known a priori how they would work—turned out to be good for gene engineers of the 21st century.
The one frustrating thing is because of crazy wokeism, and fear of crazy wokists, the most interesting phenotype of all is lagging because everybody's afraid, even though there are very good reasons for medical researchers to want to know the cognitive ability of people in their studies. For example, when you want to study aging, or decline of cognitive function memory, in older people, you want to have baseline measurements of how good their cognitive function was when they were younger, right? So very good reasons for why you want to have all this data. But, researchers are afraid because it's also linked to all these controversial social issues. So, there's just a ginormous amount of genomic data, where there's no cognitive measurement attached as a field to that data—which would have been very cheap to measure.
Again, wokists hate this, but I can measure your IQ on a 12-minute test no problem, right? Not with perfect accuracy, but I can get instrumental measurements. If I take it the NFL has this thing called the Wonderlic—every player being considered for the draft is asked to take this Wonderlic—it's a short test, 12 minutes long, and it's pretty highly correlated (0.8 or 0.9), maybe with a more fulsome IQ measure. So, it would be trivial and inexpensive to gather this data. Once we have my prediction from this earlier math that I was talking about, when you get to a border a million, it could be 1 million or 2 million well-phenotyped people and genomes, we would be able to build a pretty decent IQ predictor that might have a standard error of maybe 10 points or something. That would be incredible for science, but not getting done.
Dwarkesh Patel 1:14:58
Suppose there are differences in how things are tagged between different ancestral groups (I'm not talking about average differences or anything, just how the genotype is tagged). And if the Chinese do this first, then, they have an advantage that can't be transferred over, right? Because it's only applicable or advantageously applicable to their population.
Steve Hsu 1:15:24
That's a great point. Even a small country like Singapore or Taiwan has enough data to do this. No problem for Estonia. They could do it and have this thing working and just not share it with anybody. So, it's certainly possible. Now that's a little bit too science-fictiony because the leaders who run these countries are not transhumanist rationalists people who read your blog or my blog posts on the internet. I don't think anything that exciting is going to happen. Maybe it will.
Tradeoffs to intelligence
Dwarkesh Patel 1:15:59
Do you think that the potential for pleiotropy is higher with intelligence? I mean, with certain populations? Oh, of course, by the way, they're slim or 5000 is not enough, blah blah blah. But given that you see with certain populations like Ashkenazi Jews, you have a higher incidence of nervous system disorders like Tay Sachs and other things, that seems potential to be the trade-off of higher average intelligence. Do you think that maybe pleiotropy has a higher chance of occurring with intelligence?
Steve Hsu 1:16:39
It can only be speculation at this stage. With the history of the Ashkenazi Jews, they also went through some very narrow population bottlenecks. There are some special aspects of their genetics. Whether it's related to cognitive function or not, we don't really know for sure, but there are many reasons why they have a fairly high proportion of inherited diseases and things that they're dealing with. This is one of the reasons why Israel is so progressive when it comes to genetic screening and IVF.
One thing people talk a lot about is schizophrenia. So they say that schizophrenia could be correlated with creativity. So if your brother's schizophrenic, maybe you're more likely to be creative. He's super creative, but we don't know what he's talking about. Hahahah. So people say that if you start screening against schizophrenia, maybe we won't get creative geniuses. So there are all kinds of pleiotropic things that are possibly true. But the thing I keep wanting to go back to is that if it's 10,000-20,000 different genetic variants, locations in your genome, that are more or less determining your genetic, cognitive potential, I can go around the high dimensional space. If I find out you can make someone smart using this stuff in this cluster, but it makes them dull or makes them autistic, or it makes them they don't have big muscles, I'll just go round. I don't need to use those, I have plenty more, look over here! Those 500, I don't need to use, I will use *these* 500. This is why it's important to look at historical geniuses who were pretty normal. Maybe they're even good athletes. And, maybe they even were good with the ladies. These people existed. So you have these existence proofs that I can if I need to, if I'm a really good genetic engineer, and I can operate in this 10,000-dimensional space, whatever obstacle you put for me, I will just drive around it. I need lots of data and lots of ML. I'll do it. That's the answer, which, again, most people don't really get. But it's true.
Dwarkesh Patel 1:18:56
So I mean, there's a thing where if two traits are correlated at the ends, that person who was, for example, the smartest will not necessarily be the person who is strong. These aren't necessarily correlated, but the person who has the highest mathematical ability will not be the person who has the highest verbal ability—even though the two are correlated.
At some point, it'll be interesting because parents will have to make that trade-off, even if two things are extraordinarily correlated. It'll be interesting to see how they choose.
Steve Hsu 1:19:21
Eventually, you'll have to trust your friendly neighborhood genetic engineer to advise. There's gonna be a lot more modeling going on in the background.
Dwarkesh Patel 1:19:30
For the time being, we're stuck with educational attainment as a correlate. That concerns me because educational attainment also probably correlates with other things that somebody might want or they might not want—which are conscientiousness and conformity. If you're Bryan Caplan Caplan, in the case against education, he says that the three things education signals are conscientiousness, conformity, and intelligence. You want intelligence? Most parents probably want conscientiousness rather than conformity, but some might not. Hopefully, we can get the direct intelligence data itself. But, is there some way to segment out the conformity part of that educational attainment data?
Steve Hsu 1:20:12
In my dream world, if I were the CEO of 23andme or something, what would I do (oh, warning, they're actually secretly doing this, but you didn't hear that from me)? I would have little surveys on the site that say, “Can you do a personality survey, and one of the categories will be conscientiousness, and one will be extraversion, right?” Conformity is not a traditional, Big Five thing. But, you can have questions about how conforming someone is. Of course, we know how to do a little math. So, we can diagonalize a matrix of correlated measurements of all these different things. So, I might be able to remove the chunk within EA (Educational attainment), which is due to conformism, remove the chunk, which is due to conscientiousness, and leave behind the chunk, which correlates highly with the separate IQ predictor that I built separately using a different method. All these things are understood solutions, these problems are understood. It's just a data problem.
I'll tell you an interesting thing. There are 20,000 sibling pairs in the UK Biobank. Three years ago, most people didn’t really understand these polygenic scores, and they were very skeptical, thinking that we weren’t really capturing the real stuff, etc. My group was the first to say: let’s look to see how well we can predict which of the two brothers who experienced the same environment will be taller. How well does my predictor do that? I'm going to predict which of these two brothers has diabetes. Now does the diabetes predictor really do that? You're modeling out all the environmental shit because they grew up in the same family, right? We showed that the predictive power falls off if you're trying to do this trick with unrelated pairs of people versus brothers who grew up in the same house or sisters is minor. It's a small fall-off in predictive power.
Basically, we are getting the true genetic stuff. One of the interesting things is when you look at EA— if you build a predictor and you ask, “Does it work better or worse when I try to predict which of the two brothers got more education?” It turns out it works much worse. And that’s because part of what that predictor is capturing is some maybe property of the parents who beat them and made them go to school, but both brothers got beaten and had the skill—so that the reduction in quality of EA prediction for brothers is quite a bit higher than if you're just trying to predict G (General Intelligence).
So we have predictors we built that just predict G. Those have a much smaller reduction in quality when you apply them to brothers than in unrelated pears. I went through that quickly, so people could look up the paper. But the point is that we can see EA is weird and is a very different trait than G from these kinds of results. Again, people who criticize us have no idea how sophisticated the work is. They don't read our papers.
If they try to read our papers, they can't understand them. But we've done all this stuff. Now, a guy who comes from a physics background or from an AI/ML background can absorb it. But a lot of our critics just can't absorb it. It's literally a G thing. They can't absorb it. So they just want to keep criticizing us forever.
Dwarkesh Patel 1:24:04
The funny thing is that I have a much easier time when I read your papers. In the pros part. And the explanation in the organization is…I don't know if it's your physics background, or whatever. But, I noticed it's Scott Aaronson’s papers as well. They're written like essays, as long as you understand the underlying ideas, they're so easy to absorb. Whereas, if I just read a random thing on Bio Archive, it’s like “I don't even know where to get started with this.”
It is just written so turgidly.
Steve Hsu 1:24:30
I'm totally with you. There are multiple reasons for this. One thing is maybe that I'm an outsider. So I'm trying to write it very clearly. Conceptually, maybe the theoretical physicists would write it. But also it's a slightly selected population. Scott has an enormously popular blog, and he writes these huge posts all the time. I have a blog too, so we are a little bit better at expressing ourselves or clarifying ideas than the average scientist who's just trying to get the thing out and publish it in Nature.
Consumer preferences
Dwarkesh Patel 1:25:01
Awesome. Let's talk a little about what consumers actually want. Gwern has this really detailed post about embryo selection. He writes in it, “My belief is that the total uptake will be fairly modest as a fraction of the population,” and he's talking about embryo selection there. “A large fraction of the population expresses hostility towards any new fertility-related technology whatsoever, and people open to the possibility will be deterred by the necessity of advanced family planning and the high financial cost of IVF, and the fact that the IVF process is lengthy and painful.” So, he seems very pessimistic about the possibility that this is something that millions of people are using—what is your reaction to his take here?
Steve Hsu 1:25:49
There are two perspectives that you could adopt in looking at this. One is a venture capitalist perspective, where you ask: “How big is this market? What's it worth dominating this market? What valuation should I accept from these pirates at GP?” The other perspective is being worried that humans are all going to engineer themselves to be blond, 6’4, and we're going to be suddenly susceptible to all kinds of diseases—and one single, cold virus will kill all of us. So, there's two different perspectives on what level of penetration will this technology have.
From the venture guys' perspective, I will just say this: one out of 10 babies in Denmark is born this way. Would you capture a market that interfaces with one out of 10 families, and that's going to grow, of course. One out of 10 families in all developed countries, maybe including China. Do you have the genome of mom and dad and the kid? Maybe you can sell them some health services later on? Maybe your relationship with these people is sticky? That's for the venture guys. From the, “Oh, I'm really worried about human evolution!” Or, “When are we going to get another von Neumann?” That's a different question.It may be that it'll never be more than 10 or 20% of the population that's using IVF. Through IVF, embryo selection, and maybe potentially editing someday. In that sense, why worry, there's always going to be this natural reservoir of the wild type that has much more genetic diversity? Maybe this is the Goldilocks world. But imagine the Goldilocks world where there's plenty of wild-type people, and then there's plenty of people using these advanced technologies, and everybody's happy—including our investors.
Dwarkesh Patel 1:28:01
Something tells me that that will not be satisfying for the people who are concerned about the evolutionary diversity or whatever. I have the sense that this whole argument is just a front for a moral reservation about this technology.
Steve Hsu 1:28:17
Exactly. It's a front for people who just hate it. But what is Gwern saying? Is he saying that these 10% of babies born in Denmark are already mostly screened for chromosomal abnormalities? If I take that same data, and I can generate this other report, are you really not going to look at that report? Are you gonna say, “Well, one of my one of these kids is going to be super high risk for macular degeneration or something, but I'm already screening them for chromosomal abnormalities? Is that really going to happen? I don't think so. That 10% of the population that's using IVF is going to look at the report, which can be generated by the cost of running some bits through the AWS server.
I'm not sure what he means by that. I admire Gwern a lot. But what does he mean by that? Not many people are going to adopt it. Does he mean that the percentage of adoption within IVF families or the fraction of the population that's already doing IVF? Because those are already big numbers. So I don't know what he means.
Dwarkesh Patel 1:29:28
One way to think about genetic prediction, given your earlier statement about the Scandinavian countries doing a lot of IVF, is that it’s because of how old people are when they're having babies. A venture capitalist can think of your company as a way to get exposure to demographic collapse, right?
Steve Hsu 1:29:47
Yes, that's been mentioned. By the way, it's 3-5% of us. It ain't small. If you go to a kindergarten, there are IVF babies there. Have you seen IVF babies running around in the playground? So I don't know whether their perspective is, is this a big enough market for you to make money in it? Or is this going to change the future of the human species? You can have different perspectives.
Gwern
Dwarkesh Patel 1:30:14
By the way, Gwern is such an interesting character. I've been reading him for a long time, but obviously, his persona is very mysterious. Do you know what is going on here? How did this person get into it? It's a really interesting and detailed report he published in every selection. What is going on here?
Steve Hsu 1:30:40
Well, Gwern is a super smart guy! I know a lot of scholars and serious scientists and intellectuals in the academy, and even though I didn't quite agree with his take that you just mentioned–– I mean, it might not be technically wrong, because I'm not sure what he meant by his words. I'm not even sure he would disagree with the quantitative things I just mentioned to you. But, I just want to say some positive things about Gwern because I like to read his stuff.
In the early days, he was already following much of this stuff about genomic prediction and embryo selection. He's written stuff on GPT-3 and alignment risk. He's written lots and lots of insightful things.
He's quite impressive, even if you compare him to the most, famous academic scholars—whether it's Steve Pinker, or somebody who has written a lot of stuff that people read—and as obviously, been thinking deeply about a lot of different things during the course of a very serious life. I think Gwern is super awesome. He's right up there with those guys. So, it's awesome that we live in this internet age where some totally anonymous dude can produce really good thoughts about a wide variety of things. He's not wrong with most of the stuff he writes about embryo selection—it's pretty much right. I have a very high opinion of Gwern.
Dwarkesh Patel 1:32:13
It's interesting with people like Gwern—it's almost in the model, you can think of early 20th century or late 19th century, these gentleman scholars, who would just pontificate about a lot of different subjects—I wonder if we're gonna see a return of the sort of generalist thinker. Maybe we've over-indexed on specialists, but now it's now the time for somebody like you! Theoretical physics, bringing all of that computational and mathematical knowledge to genomics—is that the new trend in science, at least at the upper levels?
Steve Hsu 1:32:47
I don't think it's a trend. So, in terms of Gwern having a platform, you can tell he's thinking and reading a lot. He's thinking, and then he's writing very insightful stuff, and he has an audience, thanks to the internet, so people can read it. That is an amazing positive trend, which will continue. So, we're in a way, in a golden age for intellectual exchange. Even the conversation that you and I are having is an example of that.
The thing I'm afraid is not going to happen is that because science is so specialized now (it takes so much money and resources and institutional support within a university or lab or something to get stuff done), it's getting less and less common to find polymathic people who can do things at the frontier, where they make a significant contribution, and it's recognized by the natives in that sub-specialty that's becoming rarer. It was much less rare in the time of Feynman and von Neumann and people like that, just because the science was smaller. Feynman played around with some molecular biology, which was a big thing. He was friends with Francis Crick—who was down in San Diego. So, he would do stuff like that. Now, it's almost impossible. People would tell me, “Steve, why’re you fucking around with this stuff? You're wasting your talent.” So, I don't think the trends are good for that. But for general intellectual exchange, the trend is good.
Will parents matter?
Dwarkesh Patel 1:34:35
Yeah, that's interesting. Going back to IVF, do you think the gains will be greater in any given trait you could think about for parents who are already high in that trait or for parents who are lower in that trait compared to the average population?
Steve Hsu 1:34:52
I don't think the base level of mom and dad is a big factor. The big factor is how good are your predictors? And how many embryos are you looking at? Or how good are your editing tools? By the way, I just want to reinforce something I recently learned—it was so impressive that it freaked me out. I thought, “Oh, I'm in this field. So in this industry, I know about it,” but our company was having some conversations with a company that handles egg donation. So it's IVF space. And the egg donors are typically young women 22-23, that could even be college-age women who are paid a sum of money to go through an IVF cycle and just donate the eggs to some billionaire family or whoever wants the eggs.
And I was told that 60 to 100 eggs per cycle is not unknown. It's shocking because usually, it's an older woman in her 30s, or 40s, who is going through it, and they're struggling just to get some viable embryos. And then, when you run that same process with a 19-year-old, what do you get? And I was shocked at how high these numbers were.
In principle, let's just imagine you're a billionaire oligarch, but very tech savvy. You want to have some, you want to have a large family, and you want to have high-quality kids, maybe very long-lived healthy Kids—you might be selecting the best out of hundreds. There are 100 parallel universes I could live in. I get to peek into each one and then choose. I'm going to step through door number 742 because that's the outcome I like. Not that expensive. But amazing that people can now do this.
Dwarkesh Patel 1:36:53
That'll imply that the returns of being young when you have kids will increase because IVF is theoretically supposed to help kids when you have kids when you're old, so it's evening the playing field. The addition of this with the additional embryos for somebody as young as I know, it's we're tilting away in favor of young now—at least of your care about those traits that genetic screening could help you figure out.
So let me ask you about what you think about some possibilities Gwern talks about in that post. One is that we might turn induced pluripotent stem cells into embryos, and then we'll be able to select across hundreds of embryos without having to harvest eggs.
Steve Hsu 1:37:41
Yeah, so eggs are the limiting factor; sperm is cheap. The stem cell technology takes a skin cell and revert it to the pluripotent state so that it can become some other cell that is a skin cell that may be an egg cell—that technology has been more or less mastered for mice and rats. There are a few labs in Japan where they seem to have fully mastered this for multiple generations of rats using induced pluripotent C to make the eggs. So, my guess would be to get it working in humans is not that hard. It's a matter of some years of just slaving away in the lab to get it working. I know of startups that are working on this. Now, there's going to be some trepidation. Initially, why would you do that if you can pay some 19-year-old to be your egg donor?
For example, some gay couples really want to do it, because maybe they think they can make their partner’s skin into an egg. So there are reasons why you do it. But for many people, they would say that an egg was made through a new and untested process and they’d rather have a normal egg. Well, I don't have that additional risk in this whole thing. So I don't know what will happen there adoption-wise. But I do think that it's just a technological prediction. It will be possible. We're not that far from being able to do it.
The fact that we can do it in rats means we're not too far. It could have enormous implications for natural selection. If you wanted to be able to select from the best of 1000 embryos, eventually there's no technical barrier. Now, I would say that on roughly the same timescale for the pluripotent production of eggs to mature, they are to be tested so that people are confident in it. Multiplex, very accurate CRISPR-based editing will also arrive on that same timescale. At that point, why are you fooling around this? I just went in and did it to make the changes I needed to make. Over that same timescale, it's roughly the timescale over which we'll figure out where the real causal is.
Dwarkesh Patel 1:40:32
Which is what is nice because, otherwise, you're just changing the tack.
Steve Hsu 1:40:36
So, all of this is stuff that I'm fully confident you're going to see. I may not see all of it, but I'll see the technology perfected; I won't necessarily see this impact on society. But you'll probably see.
Dwarkesh Patel 1:40:54
I'm hoping it's ready by the time I'm ready to have kids—which is still a while away. Another possibility that Gwern discusses is iterated embryo selection, which you can just keep…I'll let you describe how it works. But what do you think about this possibility?
Steve Hsu 1:41:08
Yeah, so you make a bunch of embryos, and then you decide which ones you want. Before you actually make it into a person so that then that person grows up and reproduces, you reproduce just using iterations of embryos. That's also plausible, too. All of these molecular technologies have a chance of working. I don't know anybody who's spending all their time working on that. But yeah, that could work as well.
Well, I still want to say that I made these jokes about the wokists, and progressives and people who hate us, and I feel it's wrongheaded of them. I consider myself a progressive, I don't consider myself woke. But the goals of having healthy beautiful people who live to be 200 years old—who’s against that? I'm also against inequality in society. Consistent with growth and advancement in science and technology, we should try to have a fairly egalitarian society. I'm for all those things. So if you're a wokeist watching this interview to just hate Steve Hsu or something, think why you're angry at me.
I'm actually exploring how the world is. Don't you want to know how the world actually is if we have an inequality problem because some people don't do well in school, don't you want to give those families these resources so they can fix it for the next generation? Isn't that the ultimate goal of what you want?
Dwarkesh Patel 1:42:50
To steel-man a little bit, someone might say, “Listen, one of the things that prevents a runaway divergence between families over time in the model of Piketty, or something, is a reversion to the mean. I listened to your interview with Gregory Clark, where he says that this is already the case. But to the extent that it doesn't get magnified over time. The reason is that it's hard to maintain a leech in genetics is because of reversion to the mean.
If you can keep that up, and if there's increasing returns to having good genes, because you can then afford these kinds of treatments, then the possibility of society, instead of a normal distribution for society, you can have a bimodal distribution that keeps getting further and further apart. That is a potential possibility.
Steve Hsu 1:43:43
The Morlocks and the Eloy. That is a fair concern that this could lead to grotesque, huge inequality. That is a risk of the technology in it. A lot of that depends on society, too. I mean, when someone confronts me with that, I will acknowledge it as a legitimate concern. But then I'll say that we live in a country—which is the richest, in some sense,—the richest country in the world, and there are plenty of people who don't even have health care. Did you worry about that inequality? We have a lot of inequality, there are a lot of things for you to worry about when it comes to inequality.
Dwarkesh Patel 1:44:26
Actually, maybe this might not be globally beneficial, both for at least this particular debate. It might be beneficial if the case was when I asked you, “Oh, do people who are lower on some trait have greater potential for increasing that trait than somebody who's higher up on it?” If that was the case, you could just say, "Listen, the smart people are just going to ask them taught at some point, whereas the dumb people can just catch up over time, right?”
Steve Hsu 1:44:51
Well, again, if you're more of a left guy, and you like government intervention, and so this becomes part of the government health care system, and it's free. You say we will allow more aggressive edits or more embryos to be produced.
For below-average families, there's a very natural way you can redistribute, just you're going to forcibly take a bunch of money from me when I die that I would rather pass on to my kids, you're going to forcibly take it from me. But you can forcibly give more genomic prediction sources to people who need them. It's easy.
Wordcels and shape rotators
Dwarkesh Patel 1:45:25
Just to shift topics quite a bit here, you had an interesting post on that recent Twitter viral meme about the wordcels and shape rotators. About how the content of a shape rotator combines two separate abilities, math, and spatial ability, that is, yeah, when you do principal component analysis and psychometrics, they turn out to be different but correlated. As a programmer, I'm really curious about which of those is the one that is required more for that particular skill set. Because I'm the type of person that when we're talking about abstractions, data structures, and the flow of a program, I just intuitively think about it. I just think and imagine what it looks like visually. Whereas I know friends who I said, “So clearly programming is visual-spatial ability. And they said that actually, they don't imagine it visually at all that for them, it's much more of just looking through the loop and asking what's going to happen next. So yeah, I'm curious, which of these is a better description of programming?
Steve Hsu 1:46:33
Your description captures the whole story that people are very different in how they attack, even though they're attacking the same problem in the way that their brain does it. That's one of the most fascinating things about this field of psychometrics in psychology is really trying to get into that. One of the things that fascinated me when I was being educated and going through training in theoretical physics and math was looking at how my classmates at Caltech or Richard Feynman, or how somebody approached a problem, which might be totally different than the way I would do it, or the way that we would communicate about the solution once we got it.
And there are clear visual people: Feynman was a very visual thinker. Other people are more logical or go verbal, where they're stepping through things. And it might even be, and they hear the arguments as they step through it or something. So everybody's different. And those things are super fascinating. Something that's gone out of fashion now, but was very, in fact, very, in very standard when I was growing up is when I took shop class, I don't know if you had to take shop class in junior high, or high school, but we had to take shop class, which where you go to bend metal, and literally they have machines that would weave I made an ashtray or something out of steel or something. So yes, in that class, which is very spatially loaded, you could have guys, I had a friend who was you ever had a very high LSAT score and went to Princeton, to study English, that guy could not spatially rotate at all, he was totally lost in figuring out how to, do the bends to make the ashtray or whatever, right.
So you see that very clearly. And in those old days, when things were more based on when you went shopping class, sometimes we just gave you a standardized test, which was a standardized test of spatial ability. So we're all, my generation is, you don't have to lie to me about all these things. We saw how it works—we saw people take the standardized test for spatial ability, especially in visualization. We saw people try to fucking work the machine, the metal bending machine, and some people just couldn't actually make the thing.
In the real economy of atoms, grams of steel, kilograms of steel (which is all moved to China now or something), all this stuff is super important. You can't just theorize about, “Okay, then I have this module that does this, and this function will have these types.” Like, well, that's nice and super valuable in this part of the economy (academia), but somebody's got to get this plant working. And it's got to be efficient. And we got to put the machines here so we don't have to carry the ship too far from here. That's very spatially loaded, which used to be part of the American economy and education system. Now it's all gone. But it's real, it's not fake. No, people are not making this up. And psychometricians of the 1950s and 60s would have been, yeah, here's my 10 volume treatise on spatial, measuring spatial visualization ability or something.
Dwarkesh Patel 1:49:43
If you read the biography of somebody like Einstein, I mean, he was especially known for being a spatial thinker. He was incredibly visual. Thought experiments like “what does it look like or what does it feel to be moving at this speed” or whatever? Super interesting. In the case of programmers, I'm not sure I got your answer. But what do you think is the more important skill for that particular discipline?
Steve Hsu 1:50:09
People are going to do it in different ways. Yeah. I do think that if you compare the category of engineers to the category of software developers, engineers generally, have higher, on average higher spatial ability, and they're using it. Whereas you can be an awesome programmer with zero spatial ability. That's my guess.
Dwarkesh Patel 1:50:31
Yeah, I wonder if when you're studying history or something, you notice that some people are really attracted to the military history aspect of it, and seeing how the units move. I wonder if that's because they have a higher spatial ability, and they need to be able to understand how the units are moving, and so on.
Steve Hsu 1:50:50
I was gonna say - this is a very weird thing for me to reveal that, sometimes when I'm having trouble falling asleep, I'll be visualizing. Recently, I was thinking about how I would use a ballistic missile to target an aircraft carrier. Or sometimes, if I'm trying to go to sleep, I'll just be visualizing, “Okay, when you're at about an altitude of five kilometers, what can your radar see? And how much resolution do you need? And then how much time do you have to hit the ship?” And, I'll be thinking about stuff for relaxation.
This is highly visual but also quantitative because you have to make some estimates. But, that would be typical of a lot of physicists. Because if we start talking about it, we'd be like, “Oh, yeah, right. And you've only got about a point of order a 10th of a second to do this. And you've bet you're thinking of doing it in milliseconds, so we're okay.” And then anyway, that type of thinking is very prevalent amongst certain types of people.
Dwarkesh Patel 1:51:52
Right. Now, I'm curious why it's the case that people from physics often transition to finance. I know that was something you're considering at one point—is the underlying knowledge of mathematics just the same? Or is it just such a credible signal of mathematical ability? And gee, do quant firms want to hire physics students?
Steve Hsu 1:52:20
The answer is a little bit complicated. All the factors you mentioned are true. But one of the things was that in the early phase, in the 80s, and 90s, when a lot of people in my generation went into finance, a lot of them went to trade derivatives. If you look at options, pricing theory looks a lot like physics—it's the mathematics of random walks. So, there was a very tight, not tight connection. But the concepts were strongly related to what was necessary. Now, he brought it out a little bit more to say, “Okay, but nowadays, if you go to really big quant funds, and they're looking for a signal and analyzing tons of data,” they're not trading derivatives, just actual names of stocks or whatever.
There's more load on people with machine learning and CS backgrounds now. The physicists who go in there have to use that subset of their skills, but the funds would just as soon hire a CS or ML-type guy to do it. So it's a little bit of a complicated answer.
Dwarkesh Patel 1:53:28
Yeah, that's super interesting. Because I mean, back in the 90s and early 2000s, I read that book about the fall of long-term capital management.
Steve Hsu 1:53:42
Actually, there are two books; there's one called When Genius Fails. And then there are actually three books, at least three books, but they're all good.
Dwarkesh Patel 1:53:50
Then you just hear about the people who created options and pricing theory there, about applying calculus to random walks and stuff—stuff I don't understand. But just super cool that you have these mathematicians that are just coming in and applying these ideas to finance.
Steve Hsu 1:54:06
They will. I want to say one thing about physicists, which is a little different from mathematicians, and computer science guys. Maybe not so different from data science guys, but definitely different from most computer science guys and most math guys because we spend a lot of time looking at bad noisy data. So even if you're a theorist, you had to go through these lab courses. For me, those lab courses were among the hardest, the worst! You’d have to go in and build some electronic equipment to take some data, and it could be extremely noisy. You're measuring muon cosmic rays coming through the roof and hitting your detector and then, you have to analyze the data.
And, when you're building this thing, you screw it up. You get data that makes no sense, or you say something about the amplifier wasn't right, or you're used to seeing data that sucks. You have this theoretical view of what should be happening, maybe you're visualizing it as the muon comes in, and it does this and, and interpolating between the theoretical view of what should be happening with the particles and the systems, and what the actual data looks like. Saying, “Oh, shit, we didn't do this, or we didn't shield this part.
So that's why we're getting that.” That's something physicists are very used to doing. Mathematicians are often shitty at it—they just accept, “Oh, I just accept this is the data. Now I will now reason with this data.” The same could be true for computer science people. But you need someone who's actually had to deal with shitty data and try to connect it to a very elegant mathematical model. That's something physicists are uniquely used to.
Dwarkesh Patel 1:55:45
That's also true of CS people, which is that in debugging, there are many potential problems that could happen. Obviously, one of them is that you wrote the code wrong. But often you get to the actual implementation, there are so many layers of abstraction beneath you and above the actual hardware that you have to figure out like, “Why is the correspondence between this idea I had and the actual program output not the same?”
Steve Hsu 1:56:14
That's fair. Because when you debug your code, there are many different ways it could have failed. You have to, in a sense, step back and model , “Oh, maybe this module is feeding me something wrong. And that's what's causing the problem, or this other layer.” So that is very analogous to when we have to deal with a physical experiment in the lab. The thing with physics, though, is that we were really, really geared towards getting toward the underlying reality.
Like if it’s really late at night, and my lab partner and I just want to get out and go to sleep, we can't tell ourselves that things are okay. “We didn't actually screw up the shielding on that.” “It's okay, and we'll just bring the data home and look at it.” Now we have to actually decide, do we have to spend three more hours ripping this thing apart and re-shielding it?
Or we have to get to the real underlying reality, and we can't fake it. We can't just pretend that this admission scheme will work perfectly. We can't lie to ourselves about it. That's true for coders, too. But, anyway, it's very different from social scientists and stuff where they can just decide I don't like that reality, I'll just make up this model for how society behaves. And then I'm done. We can't do that.
Bezos and brilliant physicists
Dwarkesh Patel 1:57:29
Yeah. So given the theoretical physicists' skill set, as you just mentioned, is it potentially the case? I mean, obviously, the common criticism of physics as a community is that they're absorbing too much talent—that three or four standard deviations above average intelligence people are working on a field that, in popular convention, at least seems, isn't making as much progress.
Dwarkesh: More people in physics are making the step you made, which is learning all these skills in theoretical physics, and moving out of it. Maybe finance is one way in which we're getting these pro-social benefits from the skills that physics builds. Stepping into fields of genomics or things like that. Should more physicists be just using their skills elsewhere?
Steve Hsu 1:58:16
Number one, the attrition rate is super high. So even if you take this set of kids that are plus three or four standard deviations in ability, and they enter a physics major at Princeton, or MIT or something, the fraction of them that actually end up as practicing physicists is pretty small. So they're bleeding off at all points.
Bezos started in physics and, toward the end of his Princeton career, switched to computer science. Elon was in graduate school in physics at Applied Physics or physics at Stanford, and he bled out. So it's already the case that for me, one way to say it is that education is phenomenal; you should try to get that education; it'll pay off for you later. You'll probably bleed out, and you'll trade away and do something else. Now, if you say, “Okay, of the thousands of theoretical physicists, or physicists who do fundamental research, including the experimentalists, around the world, there are tens of thousands. Maybe some of those guys should also be doing more cancer research or financial modeling.”
Maybe we should tear them off, you should remove even more of those guys and have them do more so that there's still some argument in favor of that. But, we do need a core of people that are trying to do these tough fundamental answers to these hard fundamental questions about nature.
Dwarkesh Patel 1:59:38
The Bezos example is fascinating. For the people in the audience who might not know: His original plan was to become a theoretical physicist. He didn't pursue it because he noticed one of his friends was just so much obviously more gifted than him at that skill. The story they tell us is about Bezos working on a problem for many hours and making no progress before asking a friend who solved the entire problem in his mind. Basically, Bezos realized that it (theoretical physics) was not his competitive advantage.
Steve Hsu 2:00:20
I gotta add one anecdote. So, I know many of the guys in Bezos’ eating club, we also tick because we're very similar in vintage, a lot of them were late. So I know all these guys, I know all these Bezos stories. The funny thing is the guy you're talking about (guy who solved the physics problem in his mind), whose name I believe is Asana, is a Sri Lankan guy. He went to grad school at Caltech.
At one point, we met up at Caltech when I was visiting, and he was in grad school. I met this guy and talked to him about Bezos. We had other friends in common so we weren't focused on Bezos, but yeah, I met this guy in that anecdote that you just mentioned.
Dwarkesh Patel 2:01:19
That's good to know. Because that is relevant to my question. My friend and I have continually debated the importance of intelligence at the peak of entrepreneurial or engineering ability. And he uses that anecdote to say, “Oh, look, Bezos was not smart enough to be a theoretical physicist. So, therefore, intelligence is not that important. Beyond a confident, not incredibly high point. And afterward, Bezos was creative or blah, blah, blah. He was hard working.”
I don't know; my perception of the story was, “Okay, he's not intelligent enough to be a theoretical physicist. He's below five standard deviations or four standard deviations above the mean; clearly, just studying physics at Princeton is itself a testament that it's probably at least two or three standard deviations about, well, at least three.” What was the perception of those people you talked to at Princeton, about Jeff Bezos? Is it that he just was super hardworking or creative? Or is intelligence super high, just not high enough to be a theoretical physicist?
Steve Hsu 2:02:30
Yeah, this is a great topic that many people are interested in. And even among my close friends. In school, we all talk about this stuff. You have to distinguish between the very abstract intelligence—which is helpful in physics and math, or maybe computer science, versus a more generalist intelligence. Those are correlated, but they're not the same thing. So, I would say Bezos is probably very off-scale for ability to work hard, take risks, function under pressure, focus, and generalist intelligence.
Since these traits are at least somewhat uncorrelated, if you're top 10%, in each of these five, simultaneously, already pretty rare individual because plenty of the physics guys who did better than Bezos in the physics classes could not lead a company, they could not put together a presentation that would convince a venture capitalist to invest. So it's a different skill set that we're talking about. The idea that there's a unit dimensional measure of cognitive abilities is not that useful. I'm probably guilty. People say, "Wait, Steve Hsu just said that, but he's the guy most responsible for promulgating this perspective.
But it's only because it's the simplest thing to talk about. If you compress it to one general factor, it's just easier to talk about—it doesn't mean that the other components are not meaningful. We just got done talking about verbal vs. spatial vs. some more generalized mathematical talent. So obviously, it's a high dimensional, not that high dimensional, it's at least a multi-dimensional space of abilities that we're talking about. Now, the point about Bezos, which is nontrivial, though, which is directly relevant to the life experiences of physicists who leave physics and do other stuff, is that very often in an engineering setting, or a startup setting, people say “You don't know shit about that! What are you talking about?”
But the reality is people who do perform on a technical problem that the startup has to solve—in Bezos his case, it was often optimization of some supply chain thing or optimization of some sorting process or reducing the error rate. The people in the company uniformly say that when Bezos comes in the room, he will give us good feedback on the solution to this ops problem that could be better than what we said, or at least he finds the problems with what we said, or if we did an excellent job on it, he gets it right away, which is some executives might not get it right away.
So my point is that people with these super high raw G abilities generally can be helpful in these technological environments. Even if they don't have a lot of background, they can still come in and be helpful. And sometimes they can solve problems that the people who are well trained in the area are having trouble with. That is fair. But it's not fair to say there's just some unit dimensional measure of intelligence. This guy always beats this guy, this guy always beats this guys–it doesn't work that way. But it just, on some of these scales, these guides are generally more helpful than the critics would give them credit for.
Dwarkesh Patel 2:06:01
Your life story is an example of that. But, I had another experience of this, which was I recently interviewed Sam Bankman-Fried, who is the CEO of FTX, on my podcast. For all interviews, I tried to come up with questions that the guest probably had not heard before. I tried really hard to come up with questions that he might not have heard before that might have been really interesting and challenging to answer. I listen to all the interviews he’d ever done and then prayed for a long time. But yeah, if you look and listen to that interview, you'll notice how he answers these questions. It sounds like he was just talking to somebody about that. No matter how creative a question I could try to throw at him, it's just his ability to grok. All the context is explained in a way that an audience would understand. It was exceptional.
Steve Hsu 2:07:03
Being a super successful founder selects for the ability to figure out how to effectively communicate with a person according to their background. Whether it’s an investor or someone from a tech-heavy venture fund, you have to think about how they think about a problem. Founders are selected for being excellent multiband communicators across different cultures and stuff like this. It's not surprising to me that this guy would have those capabilities.
Dwarkesh Patel 2:07:54
Okay, so you are a practitioner of jiu-jitsu and other martial arts. One notable aspect of those disciplines is that you can punch above your weight, right? Royce, Gracie, and the UFC are great examples of this. Is that possible with a trait like intelligence? Is it possible that we have techniques or other ways of compensating for your analogy? Or just natural weight? What is jujitsu for fighting?
Steve Hsu 2:08:37
Great question. So, in a way, jiu-jitsu is applied physics because you're thinking about two arms and questions like “if it’s easier for you to punch and knock me out before I can close a distance and force you to grapple with me?” I do Jiu Jitsu so much because it's very rational. It's a scientific analysis of what two humans can do to each other. It's a technology in terms of what technologies people can use, amplifying their brain power. We're surrounded by it.
So, here's an exciting thing. Suppose you and your girlfriend are trying to get the answer to some question. And you're both using Google. There's an enormous variance in who immediately puts the search term in that gets the right at the top hit is the direct answer to your question, and that's very cheap, very G-loaded. But if you get good at using particular technologies or specific information channels, you can't amplify your ability beyond just what the raw capability is. So, my answer is that there are tools, but nobody uses them. There’s no dojo where you can go where they start teaching you immediately, “This, do this, this, this, and this,” and then go the guy who's bigger than you, take him down and choke them out. There isn't anything analogous to this for cognition. But I can see how people can amplify their capabilities in different ways, either more or less effectively.
Elite education
Dwarkesh Patel 2:10:23
Now, you had a blog post a long time ago about elite education. And in it, you talked about how even if you control for the SAT, the top jobs people hear about from elite schools are overrepresented. So I'm curious, do you think this is because of a selection effect, Harvard selecting based on personality? That selects for certain high achievers? Or is that something about being at Harvard that makes you a high achiever, but what is going on?
Steve Hsu 2:10:57
I researched this question pretty aggressively when I was first when I first became an entrepreneur. Because I was like, “Well, we can raise this much money, we can get these meetings with these funds. But how the hell did this guy raise $100 million for the stupid idea, what the hell?” And then I would start looking into this guy's background, I'd see he went to Harvard. So I got intensely interested in super outlier guys—how did this guy get a job writing for The Simpsons? What would I write? This other guy writes for The Simpsons, but he went to Ohio State. So he's ten layers of social networking away from The Simpsons, but the Harvard guys are not—his buddies at the Crimson.
There are multiple factors,why? Take two kids. They both scored 1580 on the SATs. One goes to Ohio State on the Ohio regents scholarship for engineering. And the other one goes to Harvard, even though the engineering school there sucks. What's the difference in their lives? Maybe the guy went to Harvard because he understands how the world works a little better than the other dude. When he gets to Harvard, he will meet many super ambitious, aggressive, smart kids. Some of those kids are children of super-wealthy people.
Some of them are children of super influential people. And all of them are trying to get ahead. They're super ambitious—they know what it means to be a managing director at Goldman or become a partner at McKinsey—they know what those things are. If you didn't know them because you grew up in Ohio, you learn them immediately. You just get a better view of what's possible in the elite sector of society from that exposure. So there are multiple factors in networking, some of these Harvard kids come from super wealthy families, some of them their dad used to play golf, the head of the fund that he's trying to get a meeting with, right? So it's all those things together. I'm not saying it's good, but I understand how the world works. I understand why this other dude can raise so much more money than I can raise or get meetings that I can get. Right. So that's how I was initially interested in this question.
Dwarkesh Patel 2:13:26
Why are China and India underrepresented massively in Nobel Prizes per capita? Even in computer science, when I would try to find papers on specific subjects, it was rare that they would come from China or something like that. And when they did, it was just that the quality was much worse than the ones I could find from a professor in the US. I'm curious why you think that is. It can't just be the population or anything that because when those researchers come to the US, they're producing stellar research; what is happening here? Why is this effect natural? And if so, what is the explanation?
Steve Hsu 2:14:11
Well, the easy answer to that question is that many of the things you mentioned are lagging indicators. So they reflect how the West was developed and had a solid scientific and engineering tradition, while China and India were desperately poor and didn't have any of that. In my own life, in the last 20 years when I visited universities in China, South Korea, and Taiwan. They had plenty of talented undergraduates, but the best of undergraduates always wanted to come to the US for their Ph.D. They went from that to some of the best undergraduates deciding to stay there–– so the researchers who are professors there are becoming world-class. But that happened only in my adult lifetime. So, you can see it's a heavily lagging indicator.
Interestingly, in my physics career, I knew several…. The Indian term was called toppers. So the people who take the exams rank every kid in the country who takes the exam, right? So, I knew guys who were number one, number two, or number five on the IIT entrance exam, but they ended up going to Caltech, or they ended up going to MIT. So, there's this massive brain drain. It's a super powerful, elite brain drain. MIT recently has just been recruiting. If you win one of these Olympians, you get a gold medal, and the informatics Olympiad or the math at MIT will try to get you to come to MIT. So, this enormous sucking of talent into the United States is excellent. But that's why when you go to IIT, even though the undergraduates are super bright, the professors are… (no offense to my colleagues who teach there), but if those professors got a bid from UCLA, the professors there would generally move to UCLA. So that's the difference. But that's gradually evening out.
Dwarkesh Patel 2:16:10
Are there any downsides to the fact that we can pay researchers or postdocs in the US less because we're partially paying foreign workers in visas? Is that just a market arbitrage that has positive externalities for the economy? Or is there some downside to the fact that native it's not competitive for native-born workers?
Steve Hsu 2:16:34
Suitable for the US, overall, on average, bad for developing countries because you're stealing their talent. It's terrible for native-born Americans who have to compete against the best brains from all over the world; so much harder for an American kid, too, to get the job he deserves at these elite levels strongly impacted by immigration. So, you have winners and losers. Whether there's a long-term problem for America... Some guys are super obsessed who comment on my blog now and then studied where all the IMO, international math olympiad winners were going, where are they what,and they claim they're seeing this huge drop off in kids who grew up in America who are not first children of immigrants, but have instead simply been here a while.
They just never win these competitions. So ultimately, you might be discouraging the native talent pool by just letting the door open and bringing in all these super talented people from outside. So there could be some second-order effects that aren’t so sound.
Dwarkesh Patel 2:17:49
Although it's interesting when you look at an industry like tech, there's a similar aspect of foreign competition being allowed in because of H1B visas, the compensation has remained competitive. Is it just because Tech is in super inelastic demand for talent?
Steve Hsu 2:18:10
Yeah. This is a little more focused on software development and ML and stuff, but if you look at more traditional engineering fields, which aren't as hot (ex. engineers at Boeing, etc.) those guys would probably say that their salaries are heavily suppressed by the existence of hungry engineers from India and China. So in the software/tech industry, because it's been so hot for so long, it doesn't feel this effect so much. So yeah it's got plenty of elasticity.
Dwarkesh Patel 2:18:42
Awesome. Okay, Steve, this was so much fun. I really enjoyed this conversation. Loved preparing for it, talking to you, and I really got to learn a lot more about this subject that I had been interested in for a long time. Is there anything else we should touch upon on any of the subjects we've covered today or failed to cover today?
Steve Hsu 2:19:00
Well, we've covered so much, and I just really think you're a great interviewer. Your questions are always getting at a key thing that many people are confused about. There's a lot of depth there. So, I thought it was great. There's plenty more we could talk about—we should just get together and do this some other time. But I don't think you left anything out.
Dwarkesh Patel 2:19:21
If you're willing, I would love to do version two of this, where we talk about your physics work and the other subjects we might have missed this time around.
Steve Hsu 2:19:29
Yeah, we get to talk about many worlds and quantum computing. Yes.
Dwarkesh Patel 2:19:33
Haha this will be fun. In the meantime, do you want to give people your website, your podcast, and your Twitter, so they know where to find you?
Steve Hsu 2:19:45
My last name is Hsu. That's the hardest thing for people because it's anti-phonetic. Just search for me. I'm on Twitter. I have a blog and a podcast called Manifold, which doesn't have a huge listenership, but I tried to keep the quality level high and get best-in-class guests. We’re willing to go into some depth, so it's got a very niche audience. But if you'd like the conversation that we just had here, you'll probably like Manifold. So you can look for that in all the usual places you get your podcasts and YouTube.
Dwarkesh Patel 2:20:23
The podcast is similar. It's exactly what I'm trying to do here. You just know so much about so many different fields. It's so fun to listen to where you're having expert-level conversations and everything from social science to foreign policy. So yeah, Manifold podcast is a place to check out.
Steve Hsu 2:20:54
Yeah, my pleasure.
Share this post